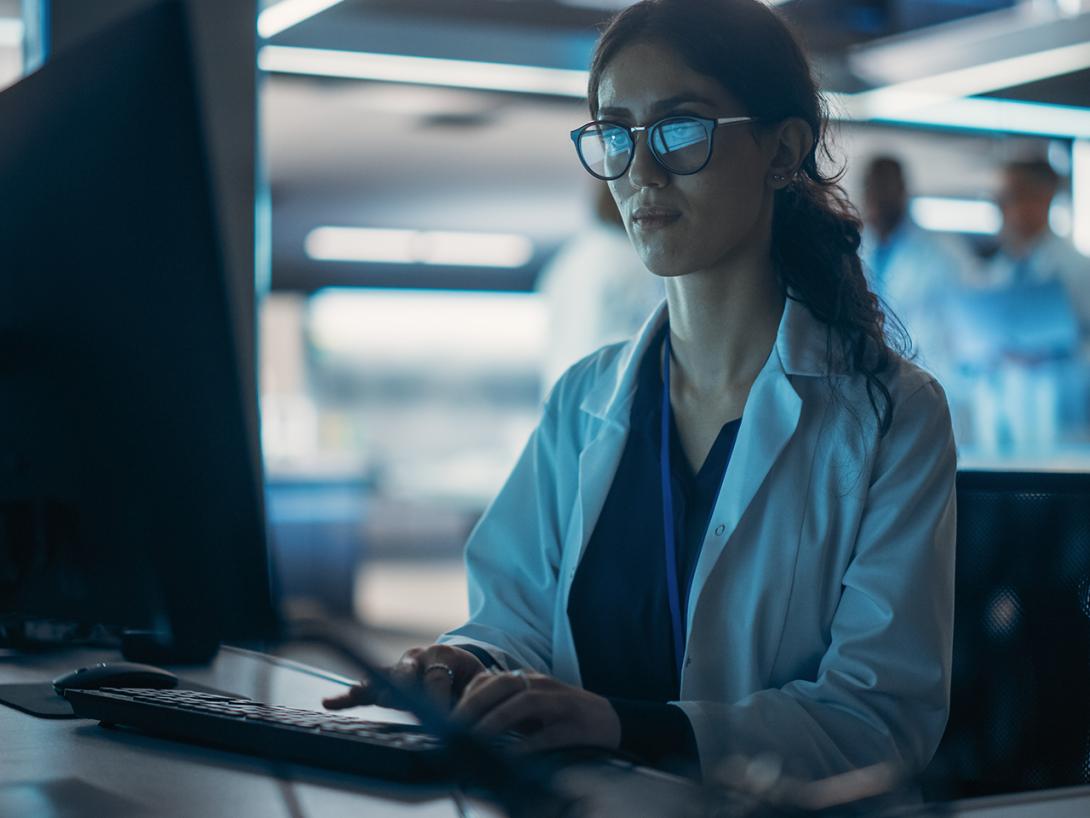
Introduction
The Agency for Healthcare Research and Quality (AHRQ) Clinical Decision Support Innovation Collaborative (CDSiC) reflects a broad stakeholder community at the forefront of using technology to support evidence-based care delivery and improve patient health and outcomes via clinical decision support. Central to the CDSiC’s mission is serving as a proving ground to design, develop, disseminate, implement, use, measure, and evaluate evidence-based, shareable, interoperable, and publicly available patient-centered clinical decision support (PC CDS). Using digital technology and tools, PC CDS aims to give patients, caregivers, and clinicians evidence-based, patient-specific clinical guidance to inform care decisions. PC CDS can be delivered to patients and caregivers—for example, through mobile apps and patient portals—or to clinicians primarily through electronic health records (EHRs).
As the use of AI grows in healthcare, including CDS, opportunities exist to improve care delivery by analyzing patient data, processing large amounts of clinical data to provide recommendations, and supporting clinical decision making with patients and caregivers.[1][2] AI can include both predictive and generative models. Predictive AI includes machine learning, statistical modeling, and data-mining techniques that support predictive analytics,[3] while generative AI involves models that can generate novel text, images, and data.[4] Within the context of PC CDS, care teams can leverage either type of AI to provide timely information for patient care, synthesize patient-generated health data to help inform care decisions, engage with patients and caregivers between visits to facilitate shared decision making, and warn of potential problems that have been shown to impact patient outcomes and quality of care.[5]
In its third year, the CDSiC developed two reports and conducted two real-world pilot projects to understand how PC CDS can better leverage AI. This Viewpoint highlights four themes with important implications for the use of AI-enabled PC CDS:
- Promoting patient trust, transparency, and explainability in AI-supported PC CDS.
- Understanding how to scale AI-supported PC CDS.
- Keeping humans in the loop.
- Testing AI-supported PC CDS in the real world.
Promoting Patient Trust, Transparency, and Explainability in AI-supported PC CDS
The “black box” nature of AI—a lack of transparency of the inputs and algorithms used by AI to generate output—is a key challenge to using AI-supported tools in healthcare.[6] Such opaqueness can lead to mistrust of AI-generated output among clinicians, patients, and caregivers, with potential downstream consequences for using AI guidance in patient–clinician interactions.[7] Discussions about AI at the 2023 and 2024 CDSiC Annual Meetings[8] identified improving patient trust, transparency, and explainability of AI-supported PC CDS as a key focus area to leverage AI effectively for PC CDS.
The Implementation, Adoption, and Scaling Workgroup: Landscape Assessment on the Use of Artificial Intelligence to Scale Patient-Centered Clinical Decision Support report identified explainable AI as a promising practice to address challenges associated with the black box nature of AI tools. Explainable AI makes the patterns underlying AI decisions clearer to researchers and clinicians—for example, through example-based explanations that accompany output, which can build trust and provide a better understanding of the reasoning behind AI-generated recommendations.[9] The landscape assessment indicated that the use of black-box AI systems should be strongly discouraged in favor of explainable AI—this is also the prevailing direction of several certification and regulatory agencies such as the Assistant Secretary for Technology Policy/Office of the National Coordinator for Health Information Technology (ASTP/ONC),[10] the National Institutes of Science and Technology (NIST),[11] and the U.S. Food and Drug Administration (FDA).[12]
As AI use in healthcare continues to grow, there is increasing awareness of the need to ensure the trustworthiness and safety of AI methods and technologies.[13][14] This is particularly true for generative AI, given its relative nascency in the healthcare field and the “black box” nature of AI-generated output.[15] To build trust, we need to understand patient and caregiver viewpoints on AI’s role in PC CDS, particularly during the design and implementation stage. The Trust and Patient-Centeredness Workgroup: Patient and Caregiver Perspectives on Generative Artificial Intelligence in Patient-Centered Clinical Decision Support report describes patient and caregiver perspectives on AI and considerations for developing AI-enabled PC CDS tools that support trust and patient-centeredness. While these considerations can apply to both predictive and generative AI models, we focus on their relevance to generative AI.
The report highlights considerations for the design and implementation of patient-facing, generative AI-supported PC CDS tools, including:
- engaging patients and caregivers when designing, developing, and implementing AI-supported PC CDS;
- providing patient education and training;
- developing standards and design principles to promote safe implementation of AI-supported PC CDS;
- conducting continuous monitoring of AI-supported PC CDS; and
- considering potential challenges related to mistrust, particularly for vulnerable populations.
Additionally, patient and caregiver advocates interviewed for the Patient and Caregiver Perspectives on Generative AI report described several factors related to transparency that influence their trust in AI technology. They noted that users should 1) be explicitly informed when they are interacting with AI rather than a human care team member, 2) understand who has access to data collected by AI-supported tools and how these data are stored and shared, and 3) receive information on the underlying evidence used to inform AI-supported PC CDS output (e.g., what data sources the tool is pulling from).
Future studies should continue to explore the impact of generative AI-supported PC CDS, particularly among different patient populations, and the effects of improved transparency and explainability of these tools on building patient trust and patient-clinician relationships.
Understanding How to Scale AI-supported PC CDS
As interest grows in using AI to scale PC CDS—defined as efforts to widen use of PC CDS across health systems and patient populations—carefully exploring opportunities, considerations, and recommendations for using AI to scale PC CDS is a critical early step.
The Implementation, Adoption, and Scaling Workgroup: Landscape Assessment on the Use of Artificial Intelligence to Scale Patient-Centered Clinical Decision Support report discusses how AI is being used to scale PC CDS, outlines key considerations for using AI to scale PC CDS, including promising practices for doing so in a patient-centered way, and discusses opportunities to advance the use of AI to scale PC CDS. The report highlights five areas where AI can be used to scale PC CDS:
- automating processes;
- facilitating the technical development and support of PC CDS;
- complementing direct or immediate clinician interaction;
- supporting cognitive processes and decision making; and
- facilitating sharing and replication of PC CDS.
Promising practices center on viewing AI as a complement—rather than a substitute—to human interaction, providing clinician and patient education on how to incorporate AI-supported PC CDS into their work and lives, and providing standards for improved reporting on tool development. Strategies to ensure patient safety and privacy focus on addressing ethical principles, addressing differences in output, and exploring the use of synthetic data to train AI-supported PC CDS and protect patient information. These findings can help PC CDS stakeholders better understand and leverage AI to scale PC CDS more widely.
Keeping Humans in the Loop
Several CDSiC products have highlighted the importance of continued human involvement when using AI-supported PC CDS. Given that the use of generative AI in healthcare is relatively nascent, there is a critical role for humans to review AI-generated output and ensure AI is incorporated in a positive and productive way for clinicians, patients, and caregivers.
For example, the Landscape Assessment on the Use of AI to Scale PC CDS identified human involvement in reviewing AI-generated output as a key promising practice when using AI to scale PC CDS. This practice is important to confirm that AI-generated output is clinically relevant to patients, since AI may not incorporate all the relevant context that clinicians are aware of. Additionally, human involvement will help catch errors caused by AI confabulations that deliver incorrect or misleading information.
Additionally, the Patient and Caregiver Perspectives on Generative AI report noted that AI should be used as a complementary tool to support and strengthen clinicians’ work. Patients rejected the notion of AI tools eliminating essential clinician involvement and cautioned against overreliance on such tools. Instead, clinicians should use AI tools to improve their practice, such as by streamlining processes and more rapidly synthesizing information, to allocate more time to engage with patients and their caregivers.
This “human in the loop” approach will be critical to building trust in using AI-supported PC CDS tools and catching potentially harmful errors unchecked by other guardrails.
Testing AI-supported PC CDS in the Real World
Few studies have explored the deployment and use of PC CDS interventions in real-world practice settings, particularly PC CDS incorporating AI components.[16] The CDSiC Innovation Center has developed, pilot tested, and evaluated two AI-supported PC CDS tools with clinicians, patients, and one caregiver: 1) Quartz and 2) Patient Artificial Intelligence-Guided E-messages (PAIGE):
- Quartz. Quartz is a prototype text-based app to help patients improve medication adherence. Quartz leverages text messaging and chatbot technology to gather information from patients about their adherence to a prescribed hypertension medication regimen.
- PAIGE. Developed by a team at Vanderbilt University, PAIGE is a large language model (LLM)-powered prototype patient communication app that can improve the accuracy and efficiency of provider responses to portal messages. PAIGE takes a patient’s question and generates one or more clarifying questions to simulate a back-and-forth conversation. PAIGE then generates a summary of the conversation, which is reviewed by the patient and can be sent to the clinician for a response.
Evaluation Results. The Quartz pilot findings indicate that more needs to be done to improve the integration of AI-supported apps into clinical workflows, ensure adequate response times to patients, and ensure clinicians have correct and complete information in one place. From a patient perspective, important considerations include ensuring app responses are more personalized and empathetic and creating a feedback loop with patients. The PAIGE evaluation results highlight the need for AI-supported communication tools to incorporate more of the patient voice (e.g., using the patient’s own words to describe symptoms, using colloquial terms) as well as integrating EHR information to contextualize patient-provided information. Additionally, further usability testing with patients with limited digital and/or health literacy is needed to better understand the impact of AI-supported tools on patients and caregivers. Currently, the CDSiC is conducting real-world testing of the Quartz app in a healthcare system to assess the usability of the tool and identify opportunities for future enhancements.
Looking Forward
The CDSiC’s AI-focused products and projects have provided useful information on how to best leverage this emerging technology to improve patient experiences with care and enable PC CDS to reach more people. Now in its fourth year, the CDSiC continues to explore and address the research gaps identified through these AI-focused projects. The CDSiC Steering Committee has discussed several priority areas for the CDSiC to consider pursuing related to AI, including implications for patient safety (e.g., by reducing AI confabulations and errors), gathering patient and caregiver perspectives, improving patient and caregiver engagement and education, incorporating patient-generated health data and patient preferences, exploring approaches to measuring quality and performance of AI in PC CDS and learning from real world implementation studies.
Stay tuned for more information on new products that the CDSiC will pursue in the coming year by reading the quarterly reports developed by the Stakeholder Center and the Innovation Center.
[1] Chen M, Zhang B, Cai Z, Seery S, Gonzalez MJ, Ali NM, Ren R, Qiao Y, Xue P, Jiang Y. Acceptance of clinical artificial intelligence among physicians and medical students: A systematic review with cross-sectional survey. Front Med (Lausanne). 2022 Aug 31;9:990604. doi: 10.3389/fmed.2022.990604.
[2] Lenharo M. An AI revolution is brewing in medicine. What will it look like? Nature. October 2023. Accessed August 15, 2024. https://www.nature.com/articles/d41586-023-03302-0.
[3] Predictive Analytics. IBM. Accessed September 19, 2024. Retrieved from: https://www.ibm.com/think/topics/predictive-analytics
[4] Toner, H. What are generative AI, large language models, and foundation models? Georgetown University Center for Security and Emerging Technology. May 12, 2023. Accessed September 19, 2024. Retrieved from: https://cset.georgetown.edu/article/what-are-generative-ai-large-language-models-and-foundation-models/
[5] Bajgain B, Lorenzetti D, Lee J, Sauro K. Determinants of implementing artificial intelligence-based clinical decision support tools in healthcare: a scoping review protocol. BMJ Open. 2023 Feb 23;13(2):e068373. doi:10.1136/bmjopen-2022-068373.
[6] Chen M, Zhang B, Cai Z, Seery S, Gonzalez MJ, Ali NM, Ren R, Qiao Y, Xue P, Jiang Y. Acceptance of clinical artificial intelligence among physicians and medical students: A systematic review with cross-sectional survey. Front Med (Lausanne). 2022 Aug 31;9:990604. doi: 10.3389/fmed.2022.990604.
[7] Balla Y, Tirunagari S, Windridge D. Pediatrics in artificial intelligence era: a systematic review on challenges, opportunities, and explainability. Indian Pediatr. 2023 Jul 15;60(7):561-569.
[8] Dullabh P, Dhopeshwarkar R, Cope E, Gauthreaux N, Zott C, Peterson C, Leaphart D, Hoyt S, Hammer A, Ryan S, Swiger J, Lomotan EA, Desai P; CDSiC Annual Meeting Planning Committee. Advancing patient-centered clinical decision support in today's health care ecosystem: key themes from the Clinical Decision Support Innovation Collaborative's 2023 Annual Meeting. JAMIA Open. 2024 Oct 23;7(4):ooae109. doi: 10.1093/jamiaopen/ooae109.
[9] Anjara SG, Janik A, Dunford-Stenger A, Mc Kenzie K, Collazo-Lorduy A, Torrente M, Costabello L, Provencio M. Examining explainable clinical decision support systems with think aloud protocols. PLoS One. 2023 Sep 14;18(9):e0291443. doi: 10.1371/journal.pone.0291443.
[10] Department of Health and Human Services, Office of the Secretary, "Health Data, Technology, and Interoperability: Certification Program Updates, Algorithm Transparency, and Information Sharing," 89 FR 1192 (Jan. 9, 2024)
[11] United States National Institutes of Science and Technology. Four Principles of Explainable Artificial Intelligence. September 2021. Accessed December 10, 2024. https://nvlpubs.nist.gov/nistpubs/ir/2021/NIST.IR.8312.pdf
[12] United States Food and Drug Administration. Transparency for Machine Learning-Enabled Medical Devices: Guiding Principles. June 13 2024. Accessed December 9, 2024. https://www.fda.gov/medical-devices/software-medical-device-samd/transparency-machine-learning-enabled-medical-devices-guiding-principles
[13] United States Food and Drug Administration. Digital Health Advisory Committee Meeting: Total Product Lifecycle Considerations for Generative Artificial Intelligence-Enabled Medical Devices. November 20-21, 2024. Accessed December 9, 2024. https://www.fda.gov/advisory-committees/advisory-committee-calendar/november-20-21-2024-digital-health-advisory-committee-meeting-announcement-11202024
[14] World Health Organization. Ethics and Governance of Artificial Intelligence for Health. World Health Organization; 2021. Accessed December 10, 2024. https://www.who.int/publications/i/item/9789240029200
[15] Reddy S. Generative AI in healthcare: an implementation science informed translational path on application, integration and governance. Implement Sci. 2024 Mar 15;19(1):27. doi: 10.1186/s13012-024-01357-9.
[16] Dullabh P, Leaphart D, Dhopeshwarkar R, Heaney-Huls K, Desai P. Patient-Centered Clinical Decision Support-Where Are We and Where to Next? Stud Health Technol Inform. 2024 Jan 25;310:444-448. doi: 10.3233/SHTI231004.