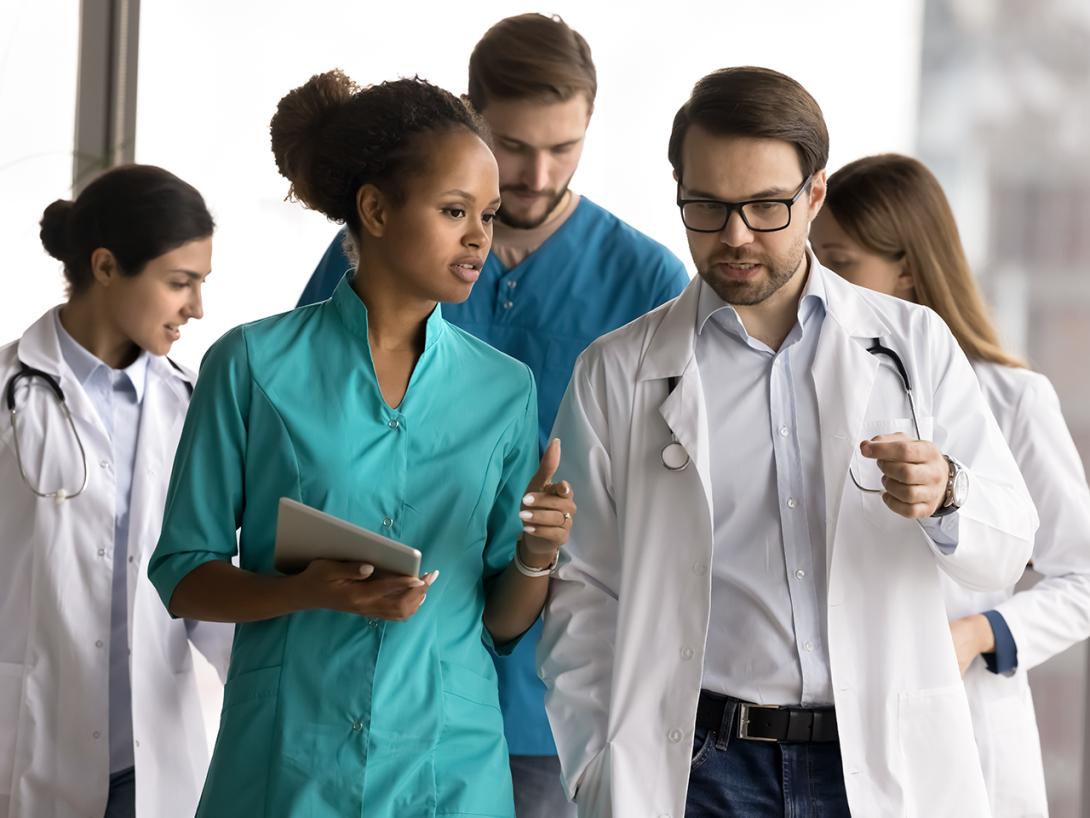
Introduction
Since its establishment in September 2021, the AHRQ Clinical Decision Support Innovation Collaborative (CDSiC) has convened four Stakeholder and Community Outreach Center (Stakeholder Center) Workgroups. These workshops developed resources to address gaps, needs, and challenges in patient-centered clinical decision support (PC CDS), as well as two Innovation Center Cores that identified measurement concepts and best practices for implementing PC CDS in the real world.
In its first 2 years, CDSiC developed numerous written products and implemented two real-world projects to advance development, implementation, and measurement of PC CDS. As the CDSiC moves into its third year, it will continue to build off the momentum of its first 2 years, as it focuses on developing a new set of products that address important research gaps in PC CDS.
This Viewpoint focuses on four overarching PC CDS themes the CDSiC is addressing in its third year, highlighting upcoming products and projects that contribute to each theme.
Exploring Use of Artificial Intelligence in Patient-Centered Clinical Decision Support
Artificial intelligence (AI) holds great potential to improve healthcare delivery. AI can process large amounts of clinical data to provide recommendations ever more quickly and accurately,[i] and has been harnessed to support clinical decision making with patients.[ii],[iii] Multiple opportunities also exist for AI to support and improve PC CDS use in healthcare for clinicians and patients. For example, AI-based tools have potential to support patients as they begin and continue to take their medications for chronic conditions—thereby reducing poor health outcomes related to poor adherence to medication regimens.[iv],[v] Additionally, large language models (LLMs) like ChatGPT can be leveraged to help clinicians respond to patient portal messages more efficiently—reducing workload challenges associated with large volumes of patient messages sent after business hours.[vi] AI also has potential to make certain aspects of PC CDS more efficient, reducing barriers to scaling PC CDS (such as high costs for creation and maintenance or time needed to collect patient-generated health data). Thus, AI holds great promise for the future.
But we are still at a very nascent phase in AI adoption and use in the field of PC CDS. As we continue to harness AI technology, it is important to identify important gaps in our knowledge of AI, as well as challenges and limitations to its use. This is particularly important regarding patient care, making it critical to understand both patient and caregiver perspectives on the use of AI within their healthcare.
In Year 3, four new CDSiC products and projects are exploring the use of AI in PC CDS.
- Landscape Assessment on the Use of Artificial Intelligence to Scale Patient-Centered Clinical Decision Support. This landscape assessment is helping us understand key opportunities and considerations for the use of AI to scale PC CDS, in particular, recommendations for using AI to scale CDS in a patient-centered way.
- Patient and Caregiver Perspectives on Artificial Intelligence. This product is describing patient and caregiver perspectives on AI, and developing a list of considerations for development of AI-enabled PC CDS tools that support trust and patient-centeredness.
- Artificial Intelligence–Based Intervention to Support Medication Adherence. This project is codesigning, developing, implementing, and evaluating a prototype text-based app to help patients improve adherence to medications. The app is leveraging an AI-based tool to have conversational dialogs via text message with patients—to ensure patients are taking their medications as prescribed, and that the medications are having the desired effect. As part of this project, the team is conducting a 3-month mixed methods evaluation of the prototype across the design, clinical use, and post-implementation phases to assess the tool’s feasibility, acceptability, and appropriateness.
- Large Language Model–Powered Prototype for Patient-Provider Communication. This project is developing an interactive LLM-powered prototype to serve as an intermediary between patients and providers. This prototype will improve the accuracy and efficiency of provider responses to portal messages, and strengthen patient-provider communication. The team is also conducting a 3-month evaluation to assess system feasibility and acceptability, and document patient and provider perceptions of the tool.
Capturing the Voice of the Patient in Patient-Centered Clinical Decision Support
Incorporating patient preferences into PC CDS ensures consideration of three important components of the patient voice: how the patient wants to interact with their clinician or data, how the patient would prefer to approach their healthcare decisions, and what healthcare outcomes are most important to the patient.[vii] In its first 2 years, the CDSiC developed a Taxonomy of Patient Preferences that identified and characterized patient preferences relevant to PC CDS. More work is needed, however, to fill knowledge gaps related to patient preferences in PC CDS. It will be important to explore what patient preference data are currently collected and measured, what data are not currently captured, and how to address measurement gaps in this area. Additionally, although some patient preferences are more commonly collected and have standardized codes,[viii] less is known about patients’ views on what patient preference concepts should be prioritized for standardization in PC CDS. Finally, while the Taxonomy of Patient Preferences product identified what preferences should be collected for PC CDS, more should be done to clarify how and when patient preferences are best captured within patient lifeflows and clinician workflows.[ix]
In Year 3, we are building on our Taxonomy of Patient Preferences to strengthen incorporating the patient voice in PC CDS through three new CDSiC products.
- Exploring Existing Measures to Assess Patient Preferences. This exploration is developing an inventory of patient preference measures and instruments—providing essential information about how the different inventory components can be used and their reliability and validity.
- Prioritizing Patient Preferences for Standardization to Support Patient-Centered Clinical Decision Support. A roundtable with patients and PC CDS experts is being organized to prioritize patient preference domains (i.e., personal characteristics, communication, access and care experience, engagement, data, and healthcare services) for standardization within PC CDS. This effort will ensure the preferences most relevant to patients are standardized and included in PC CDS tools.
- Capturing Patient Preferences for Patient-Centered Clinical Decision Support Within Clinician Workflows and Patient Lifeflows. This effort is identifying methods for capturing patient preference data in ways that not only reduce the burden for both patients and clinicians, but at the same time develop a best practice guide for capturing patient preference data in clinical and nonclinical settings.
Improving Patient-Centered Clinical Decision Support Implementation, Reporting, and Measurement
In its first 2 years, the CDSiC developed four products related to implementation, reporting, and measurement of PC CDS. The PC CDS Planning, Implementation, and Reporting Checklist supports implementation teams in detailing how they address key PC CDS implementation domains and associated patient-centered factors. Two separate products documented measures for PC CDS. The PC CDS Performance Measurement Inventory focused on process-related measures to assess the patent-centeredness of CDS implementations. The Patient-Focused Outcome Measures for PC CDS documented patient health journey outcomes that can be measured to evaluate PC CDS. Finally, the CDSiC Innovation Center developed a PC CDS Performance Measurement Framework that provides a basis for consistent measurement of PC CDS design, development, implementation, use, and evaluation across the structure, process, and outcome spectrum. All these products support PC CDS implementation teams in deciding what actions to undertake when planning and implementing their PC CDS and identifying appropriate measures to evaluate their PC CDS.
In Year 3, the CDSiC is building off this prior work by developing four resources and conducting assessments to understand how to improve reporting and measurement for PC CDS.
- Developing Rapid Patient-Centered Clinical Decision Support Planning, Implementation, and Reporting Checklists. The CDSiC is conducting a usability study of the PC CDS Planning, Implementation, and Reporting Checklist with partners. From the usability study, four streamlined, rapid-assessment versions of the overall checklist are being developed to enable users to navigate the checklist, and prioritize implementation tasks that best fit their specific implementation goals more easily.
- Prioritizing Patient-Centered Measure Areas for Patient-Centered Clinical Decision Support. This product is identifying patient-centric measures that should be prioritized during PC CDS implementation and evaluation, to focus on what is most important to patients.
- Cross-Cutting Assessment of Patient-Centered Clinical Decision Support Process and Outcome Measures. This project is testing the PC CDS Performance Measurement Framework in the real world, by examining how a select number of AHRQ-funded PC CDS grants are assessing PC CDS interventions. This assessment will provide a valuable perspective on measurement opportunities and challenges for widespread implementation and adoption of effective PC CDS.
- Case Studies of Patient-Centered Clinical Decision Support Implementations to Identify Challenges, Opportunities, and Recommendations for the Implementation, Adoption, and Scaling of Patient-Centered Clinical Decision Support. The Implementation, Adoption, and Scaling Workgroup is complementing the Cross-Cutting Assessment project by conducting case studies of real-world PC CDS implementations supported by AHRQ and others, to derive more detailed insights into challenges, opportunities, and recommendations for the implementation, adoption, and scaling of PC CDS.
Maximizing the Value of Patient-Centered Clinical Decision Support
In addition to work focused on emerging areas in PC CDS such as AI, the CDSiC will continue pursuing foundational work in three areas that represent challenges to realizing the full potential value of PC CDS. Favorable return on investment (ROI), for example, is critical to the widespread implementation, adoption, and scaling of any healthcare intervention, but there is limited literature on ROI for PC CDS, which creates a significant barrier to its widespread dissemination.[x] Additionally, many CDS systems request a reason for not following a recommendation (i.e., the override reason) to improve CDS logic and reduce alert fatigue,[xi] but there is no standardized taxonomy of override reasons for PC CDS—particularly for patient- or caregiver-facing PC CDS. This gap hinders the optimization of PC CDS recommendations across systems. Finally, although the field is increasingly recognizing the impact of social determinants of health (SDOH) factors on health outcomes, as well as the potential for CDS tools that leverage SDOH data to reduce discrepancies in health outcomes and improve clinical decision making, challenges remain to using these data in practice. Previous CDSiC work, in particular, has underscored the importance of standards for incorporating SDOH data into PC CDS.[xii]
In Year 3, the CDSiC is moving the needle in the field by developing three resources to advance the development of PC CDS in each of these challenge areas.
- Examining Return on Investment for Patient-Centered Clinical Decision Support: A Catalog of Opportunities and Approaches to Demonstrate Value. This product is providing a catalog of opportunities, approaches, methodologies, and resources through which stakeholders have sought to project or demonstrate ROI for PC CDS. By providing descriptions of example methodologies, this product will enable replicable use by those seeking to provide an ROI argument for their PC CDS interventions.
- Taxonomy of Override Reasons for Patient-Centered Clinical Decision Support Recommendations. This product is providing a written taxonomy of clinician and patient/caregiver override reasons for PC CDS. This work can serve as the foundation for creating a standard terminology.
- An Action Plan for Incorporating Social Determinants of Health Into Patient-Centered Clinical Decision Support. This product is outlining an action plan that outlines key challenges in using SDOH data for PC CDS, as well as potential short- and long-term opportunities to address those challenges.
Moving Forward
Stay tuned on the CDSiC’s product development by reading the quarterly reports the Stakeholder Center and the Innovation Center will develop in Year 3 of the CDSiC’s work.
Between now and the end of September 2024, the CDSiC will work to develop and finalize the products and projects highlighted in this Viewpoint. As our work is increasingly finalized, the CDSiC will actively disseminate our findings to a diverse audience of stakeholders—encouraging the use of CDSiC products and promoting widespread sharing of CDSiC findings.
[i] Chen M, Zhang B, Cai Z, Seery S, Gonzalez MJ, Ali NM, Ren R, Qiao Y, Xue P, Jiang Y. Acceptance of clinical artificial intelligence among physicians and medical students: A systematic review with cross-sectional survey. Front Med (Lausanne). 2022 Aug 31;9:990604. doi: 10.3389/fmed.2022.990604
[ii] Lenharo M. An AI revolution is brewing in medicine. What will it look like? Nature. 2023 October;622:686-688. https://www.nature.com/articles/d41586-023-03302-0
[iii] Ayers JW, Poliak A, Dredze M. Comparing Physician and Artificial Intelligence Chatbot Responses to Patient Questions Posted to Public Social Media Forum. JAMA Intern Med. 2023 April;183(6):589-596. doi: 10.1001/jamainternmed.2023.1838
[iv] Rosen OZ, Fridman R, Rosen BT, Shane R, Pevnick JM. Medication adherence as a predictor of 30-day hospital readmissions. Patient Prefer Adherence. 2017;11:801-810. Published 2017 Apr 20. doi:10.2147/PPA.S125672
[v] Watanabe JH, McInnis T, Hirsch JD. Cost of Prescription Drug-Related Morbidity and Mortality. Ann Pharmacother. 2018;52(9):829-837. doi:10.1177/1060028018765159
[vi] Akbar F, Mark G, Warton EM, et al. Physicians' electronic inbox work patterns and factors associated with high inbox work duration. J Am Med Inform Assoc. 2021;28(5):923-930. doi:10.1093/jamia/ocaa229
[vii] Kuperman G, Nanji K, Cope EL, Dullabh PM, Desai PJ, Catlett M, Weinberg S, Hoyt S, and the CDSiC Outcomes and Objectives Workgroup. Outcomes and Objectives Workgroup: Taxonomy of Patient Preferences. Prepared under Contract No. 75Q80120D00018. AHRQ Publication No. 23-0038-EF. Rockville, MD: Agency for Healthcare Research and Quality; May 2023.
[viii] Richesson RL, Dullabh PM, Leaphart D, Correa KH, Desai PJ, Gordon JR, Boxwala AA, and the CDSiC Standards and Regulatory Frameworks Workgroup. Advancing Standardized Representations for Patient Preferences to Support Patient-Centered Clinical Decision Support. Prepared under Contract No. 75Q80120D00018. AHRQ Publication No. 23-0074. Agency for Healthcare Research and Quality; August 2023.
[ix] Desai PJ, Osheroff JA, Jiménez F, Heaney-Huls K, Ryan S, McCoy AB, Dullabh PM, CDSiC Scaling, Measurement, and Dissemination of CDS Workgroup. Scaling, Measurement, and Dissemination of CDS Workgroup: Approaches to Measuring Patient-Centered CDS Workflow and Lifeflow Impact. Prepared under Contract No. 75Q80120D00018. AHRQ Publication No. 23-0061. Rockville, MD: Agency for Healthcare Research and Quality; July 2023
[x] Nicole M White, Hannah E Carter, Sanjeewa Kularatna, David N Borg, David C Brain, Amina Tariq, Bridget Abell, Robin Blythe, Steven M McPhail, Evaluating the costs and consequences of computerized clinical decision support systems in hospitals: a scoping review and recommendations for future practice, Journal of the American Medical Informatics Association, Volume 30, Issue 6, June 2023, Pages 1205–1218, https://doi.org/10.1093/jamia/ocad040
[xi] Shah SN, Amato MG, Garlo KG, Seger DL, and Bates, DW. (2021). Renal medication-related clinical decision support (CDS) alerts and overrides in the inpatient setting following implementation of a commercial electronic health record: implications for designing more effective alerts. JAMIA, 28(6), 1081–1087. https://doi.org/10.1093/jamia/ocaa222
[xii] Dullabh PM, Desai PJ, Gordon JR, Leaphart D, Wilson KS, Richesson RL, Boxwala AA, and the CDSiC Standards and Regulatory Frameworks Workgroup. Standards and Regulatory Frameworks Workgroup: Environmental Scan. Prepared under Contract No. 75Q80120D00018. AHRQ Publication No. 23-0029. Rockville, MD: Agency for Healthcare Research and Quality; January 2023.